
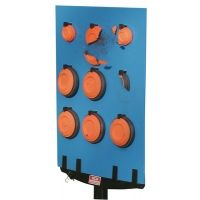
We preprocess the data before training the neural network models. Our approach provides a novel technique to classify big data sets using neural network models. We develop a neural network model to classify liver cancer patients into high-risk and low-risk groups using genomic data.

JAMMIT SITE SKIN
Relevant studies were found to show that the genetic biomarkers identified in this paper may possess value for the follow-up clinical treatment of skin cancer. Finally, gene function analysis of these 20 gene biomarkers was determined.
JAMMIT SITE VERIFICATION
A prognostic model was constructed using these 20 gene biomarkers, and the validity of the model was assessed using a training set and a verification set, which showed that the model performed well. In the present study, the least absolute shrinkage and selection operator regression analysis method was used with 10-fold cross verification to reduce the dimensions of gene data in patients with skin cancer, and subsequently, 20 gene biomarkers were screened. However, targeted gene therapy requires physicians to select the appropriate gene, which means suitable genetic biomarkers must be identified from complex genetic data. However, targeted gene therapy may be a novel means of treating skin cancer, as the targeted nature of treatment may improve therapeutic outcomes. Due to the limitations of traditional drug treatments, it is difficult to achieve the desired therapeutic effect of complete removal. Skin cancer is a common malignant tumor in China and throughout the world, and the rate of recurrence is considerably high, thus endangering the quality of life and health of patients, and increasing the economic burden and pressure to the families of those afflicted. Our numerical results showed that these 26 gene biomarkers can be used to guide the effective prognostic therapy of patients with liver cancer. The findings discovered that low-risk patients survived remarkably better than the high-risk patients (P<0.001), and the area under the curve (AUC) of receiver operating characteristic curve (ROC) was greater than 0.5. Based on the selected 26 gene biomarkers, RBM14, ALG11, MAG, SETD3, HOXD10 and other 26 genes were considered independent risk factors for the prognosis of liver cancer, and genes such as GHR significantly affect human growth hormone for liver cancer. While matching the patient samples in the gene expression spectrum, we attained 330 samples with primary tumors and 50 samples with normal solid tissue.Īfter the 330 tumor tissue samples were randomized into two equal-numbered groups (one is a training set, and the other is a test set), we selected 26 gene biomarkers from the training set and validated them in the test set. Our data were collected from a public database without real patient participation. After removing some patients with missing survival data, we collected 397 patients' samples. We downloaded the TCGA data of liver cancer on the cancer-related website (), comprising 438 patients and 20,530 genes. With the continuous advances of high-throughput gene sequencing technology and computer data mining technology, researchers can understand liver cancer based on the current accumulation of gene expression data and clinical information. Liver cancer is a common cancer that enormously threatens the health of people worldwide. This result indicates that on average JAMMIT outperformed both JIVE and PLS in detecting the two simulated signals over a wide range of SNR scenarios Note for each distribution, the area under the distribution curve is equal to one and most of this area (i.e., probability measure) is concentrated on the positive x-axis to the right of the vertical green line positioned at x ¼ 0. Each ΔAUROC distribution was based on a normal kernel smoothing function evaluated at 100 equally spaces points using MATLAB's ksdensity function. Similarly, panels b and c show ΔAUROC distributions for JAMMIT versus PLS to detect SSig1 and SSig2 in the same set of simulated MMDS used to evaluate JAMMIT versus JIVE. Panels a and b show the distributions of ΔAUROC values equal to the AUROC for JAMMIT minus the AUROC for JIVE for the detection of two simulated signals, SSig1 and SSig2, in 1000 simulated MMDS as described in the Methods section of this paper. Distribution of ΔAUROC values comparing JAMMIT detection performance with two other algorithms in simulated data.
